Using Generative AI to Produce Sentiment Analysis for Leaders in Finance

by Hagop Alajajian on Jun 16th, 2023
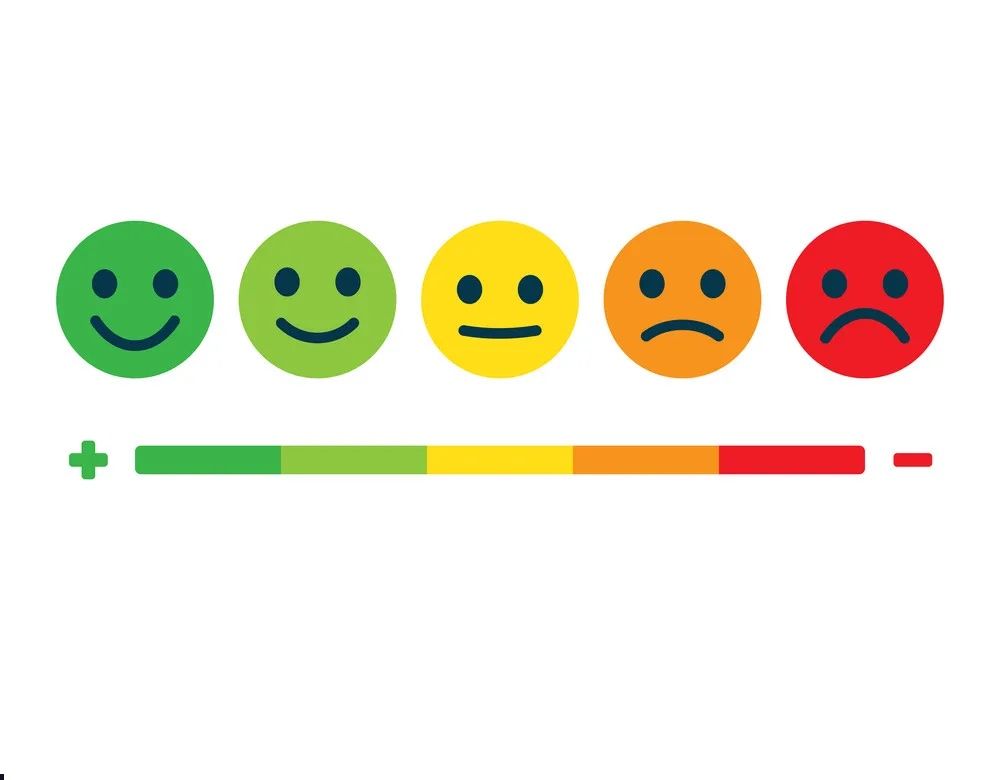
Introduction
Sentiment analysis has numerous applications across different domains. One very important transformation being made for how sentiment analysis is being conducted is through generative AI. It has become apparent that generative AI has become an extremely useful tool in many industries, and today it can be applied to the field of finance by interweaving sentiment analysis, a very powerful tool to understand emotions, trends, underlying opinions, etc. Specifically, business leaders are using to good advantage of generative AI to provide sentiments on various aspects of business, such as insight into public opinion and customer satisfaction, identifying trends in the market, among many other trends. In finance, sentiment analysis provides significant information to companies, and generative AI is making the process of conducting it much easier.
What is Sentiment Analysis?
Sentiment analysis, also known as opinion mining, is a technique used to determine the sentiment or emotion expressed in a given piece of text, such as a sentence, paragraph, or entire document. It involves the use of natural language processing (NLP) and machine learning algorithms to analyze and classify the subjective information contained in the text.
The goal of sentiment analysis is to identify and extract subjective information, such as positive, negative, or neutral opinions, as well as more specific emotions like joy, anger, sadness, or surprise. This analysis can be applied to various types of text data, including customer reviews, social media posts, news articles, surveys, and more.
How does Generative AI provide Sentiment Analysis?
Before discussing sentiment analysis, let’s realize the power of generative AI. For the first time with generative AI, software is able to efficiently and effectively provide sentiment itself. Generative models can be trained in a conditional manner, where the model is given a sentiment label as input and is trained to generate text that corresponds to that sentiment. For example, a model can be trained to generate positive, negative, or neutral text based on the sentiment label provided. Now, as you might imagine since it can generate emotion and opinions in its own responses, generative AI can complete the task of taking input and analyzing the texts’ sentiments.
As many people know with the emergence of ChatGPT, generative language models can create text based on a given prompt or context. To perform sentiment analysis, generative AI models and frameworks like ChatGPT and Generative Adversarial Networks can leverage their contextual comprehension to determine the sentiment expressed in the text. It can recognize various types of sentiments conveyed through words, phrases, or the overall tone of the content.
Relevance to Finance
There are a few major ways leaders in finance can use sentiment analysis: find out sentiments about earning calls, learn about financial news in order to predict the behavior of financial markets, insight into earning reports, and analyzing best practices amongst team members in meetings and team reports.
- Earnings call:
In the case of an earnings call transcript (which is a public company that discusses their financial results typically in a teleconference), ChatGPT can gauge the management team's confidence about the firm's prospects by analyzing the language used. It can identify positive statements about future growth, expansion plans, innovative initiatives, and optimistic projections. Conversely, it can detect negative sentiments, such as concerns about market conditions, potential challenges, or uncertainties.
Moreover, generative AI like ChatGPT can combine sentiment analysis with topic detection to extract relevant information. For example, if a company's management team discusses trends in advertising revenue extensively during an earnings call , ChatGPT can use the transcript of this conversation to recognize semantics and help analysts understand the significance of certain topics in the conversation. Based on ChatGPT’s ability to understand and output semantic analysis, it can suggest, for instance, that investors may expect changes in advertising revenue due to the emphasis placed on it during the conversation. The generative AI model then can help predict whether that change to the advertising revenue will be helpful or hurtful to the company in question.
- Financial market news:
Generative AI can determine market sentiment associated with a firm by analyzing news articles and other media sources. This capability is particularly valuable for investors, financial analysts, and traders who seek to understand how the market perceives a company and its prospects. In this process, generative AI can examine the language used by market journalists, commentators, and experts and output whether the sentiment expressed is positive or negative.
- Earning reports:
ChatGPT needs to access and extract the relevant information from the earning report. This might involve converting the document into a suitable format and removing any unnecessary sections. Afterwards, sentiment analysis techniques can be applied to the extracted text. Using the results, investors can gain insights since they will be able to identify positive trends, negative indicators, or areas of concern. For example, if the sentiment is positive, ChatGPT can highlight the why it is positive, such as revenue growth or successful product launches. If the sentiment is negative, ChatGPT can point out potential risks or challenges that might impact the company's performance.
- Team best practices
This point does not necessarily just apply to finance team leaders, but it can apply generally to the whole company. Leaders of a company can use sentiment analysis to get recommendations on how to change their company structure internally, keep track of progress, and so on. A few major ways to do this are through the analysis of meeting transcripts, meeting reports, and written work done by workers. Written work can come in many different forms: spreadsheets, presentations, code, etc. As an example, using sentiment analysis, leaders of a fintech company’s coding teams can quickly understand which team members are following the best practices and most efficient code. The same is true for leaders in finance teams that want to analyze their teams’ spreadsheets.
Sentiment analysis is important to helping businesses gain insights externally and internally in terms of finances. Applying sentiment analysis with generative AI offers a wide range of new possibilities, and the potential for generative AI to help manage the finances of companies will continue to advance.