A Comprehensive Insight into Fine-Tuning for Finance

by Hagop Alajajian on Aug 4th, 2023
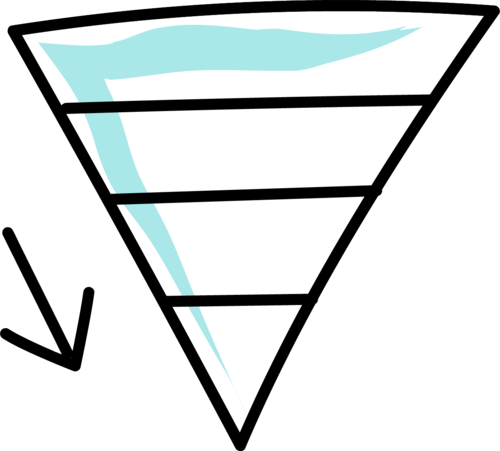
Understanding Fine-Tuning in AI
AI is a rapidly evolving field that's transforming a multitude of industries, including the investing realm. One key aspect of AI development is the concept of fine-tuning, a technique that allows models to learn from small, specialized datasets by building on the knowledge acquired from larger, general-purpose ones. In other words, fine-tuning allows a pre-trained AI model to learn and adapt to a more specialized task, even when the available data for the specific task is limited. In the realm of investing, fine-tuning has the potential to revolutionize traditional approaches, providing finance professionals with advanced tools to assess risk, identify opportunities, and enhance decision-making. Essentially, fine-tuning helps produce more specific and desired outputs when using generative AI.
Some models used for fine tuning are davinci-003 and davinci. Text-davinci-003, a modern and proficient model, is specially created for tasks that require adherence to instructions. Its design empowers it to provide concise and precise responses, even when no example prompts are presented (known as zero-shot scenarios). In contrast, davinci is highly effective when fine-tuned for a specific task, given the availability of several hundred training instances. Among other models, these can be used to help determine the most suitable model for investment-related tasks. Fine tuning these models further enhances their performance and tailors them to the specific needs of investment firms.
Fine-tuning, at its core, is a transfer learning technique. Click here to use generative AI to get a recommendation if fine-tuning is right for your task. Fine-tuning involves taking a pre-trained AI model, usually developed and trained on a large and diverse dataset, and adapting or 'fine-tuning' it for a specific task. This is achieved by continuing the training process on a smaller, task-specific dataset. The concept significantly reduces the need for extensive computational resources and time – two vital assets that are traditionally expended in the initial training process of an AI model. The technique leverages the general knowledge the model has acquired to rapidly learn the specificities of the new task. Moreover, fine-tuning allows the application of AI in scenarios with limited data availability, which is a common occurrence in specialized fields like investment news.
Implementing Fine-Tuning in Finance
In the world of finance, particularly investing, fine-tuning has immense potential for transforming traditional methodologies. Traditional investment strategies often involve human interpretation of complex financial data. However, an AI model's capabilities to analyze vast amounts of data can unearth market patterns and trends that might go unnoticed by human analysts.
One such area where fine-tuned AI models can create a significant impact is risk assessment. By training an AI model on historical financial data, we can enable it to identify patterns that may indicate a future market downturn or pinpoint assets that pose a higher risk than what appears on the surface. By integrating these AI models into the risk management process, they can serve as supplementary tools, providing valuable insights and additional perspectives that can aid in informed decision-making.
Similarly, the pursuit of alpha, also known as excess return associated with a benchmark, can also benefit from fine-tuning. Alpha is essentially the measure of an investment's risk-adjusted performance and describes an investment strategy's capability to beat the market’s edge. An AI model that has been trained to understand and interpret financial markets can be fine-tuned to identify potential investment opportunities based on a variety of factors such as company financials, market trends, and so forth. The utilization of fine-tuned AI in this context could help uncover undervalued assets and investment opportunities that may otherwise be overlooked.
Fine-Tuning AI for High-Frequency Trading
High-frequency trading is another area where fine-tuned AI can play a critical role. These trading systems involve immediate decision-making based on real-time information such as breaking news or social media sentiment. Given the need to make these decisions in very short periods of time, AI technology is well-suited for this task. Generative AI models, when fine-tuned to interpret such real-time data, can make immediate trading decisions, thereby making the high-frequency trading process more efficient.
Challenges and Overcoming Them
However, the integration of fine-tuned AI in the investment process comes with its set of challenges. Financial markets are inherently complex and often influenced by numerous factors, including ones that are difficult to quantify, such as political developments or investor sentiment. Models might fail to capture these complexities and yield inaccurate predictions. There is also the challenge of the AI model failing to perform efficiently when faced with unprecedented market events due to its training based on historical data.
Despite these challenges, the application of fine-tuning in AI technology is an intriguing and promising aspect for finance professionals. When used judiciously and in combination with traditional methods, fine-tuned AI models can help investors better navigate the complexities of financial markets.
As we move forward, it's crucial to continue refining these AI tools, ensuring they can adapt to the dynamic financial landscape and provide tangible benefits. With continuous exploration and innovation, the field of investing can evolve even further, bringing together the best of human expertise and cutting-edge AI technology.